Imagine a world where machines learn from their mistakes and adapt to new situations just like humans do. This is not a scene from a science fiction movie, but a reality being shaped by reinforcement learning, a powerful branch of artificial intelligence. Today, we’re teaching machines not just to perform tasks, but to learn and improve from their experiences. This blog post will take you on a journey to understand the basics of reinforcement learning, its controversies, real-world applications, and its potential to unravel the future of AI.
The Basics of Reinforcement Learning
Just as a child learns to walk by taking small steps, falling, and then adjusting their movements based on what they’ve learned, reinforcement learning algorithms learn through a process of trial and error. At the heart of this process are four key components: the agent, the environment, actions, and rewards.
The agent is the learner or decision-maker. The environment is everything the agent interacts with. Actions are what the agent can do, and rewards are the feedback the agent gets from the environment. The agent’s goal is to learn a policy, a strategy that tells it which action to take under what circumstances, to maximize its total reward over time.
The Controversy: Reinforcement Learning vs. Other Learning Methods
In the world of machine learning, there are several methods for teaching machines to learn from data. The most common ones are supervised learning, unsupervised learning, and reinforcement learning. Each of these methods has its strengths and weaknesses, and the choice of method often depends on the specific problem at hand.
Supervised learning is like a teacher-student relationship. The algorithm, or student, is given a dataset with both the inputs and the correct outputs. The goal is to learn a function that maps the inputs to the correct outputs. This method is powerful and widely used, but it requires a large amount of labelled data, which can be expensive and time-consuming to collect.
Unsupervised learning, on the other hand, is like exploring a new city without a map. The algorithm is given a dataset without any labels, and its goal is to find patterns or structures in the data. This method can uncover hidden patterns that humans might not notice, but it can also be more difficult to interpret and control.
Reinforcement learning stands apart from these methods. It’s like learning to ride a bicycle. The algorithm learns by doing, receiving positive or negative feedback for its actions, and adjusting its behaviour to maximize the positive feedback. This method can handle complex, dynamic environments and learn from sparse, delayed feedback, but it also requires careful design of the reward function and can be computationally intensive.
Despite these challenges, reinforcement learning has shown great promise. For example, Google’s DeepMind used reinforcement learning to train their AlphaGo program, which defeated the world champion in the game of Go, a feat previously thought to be decades away.
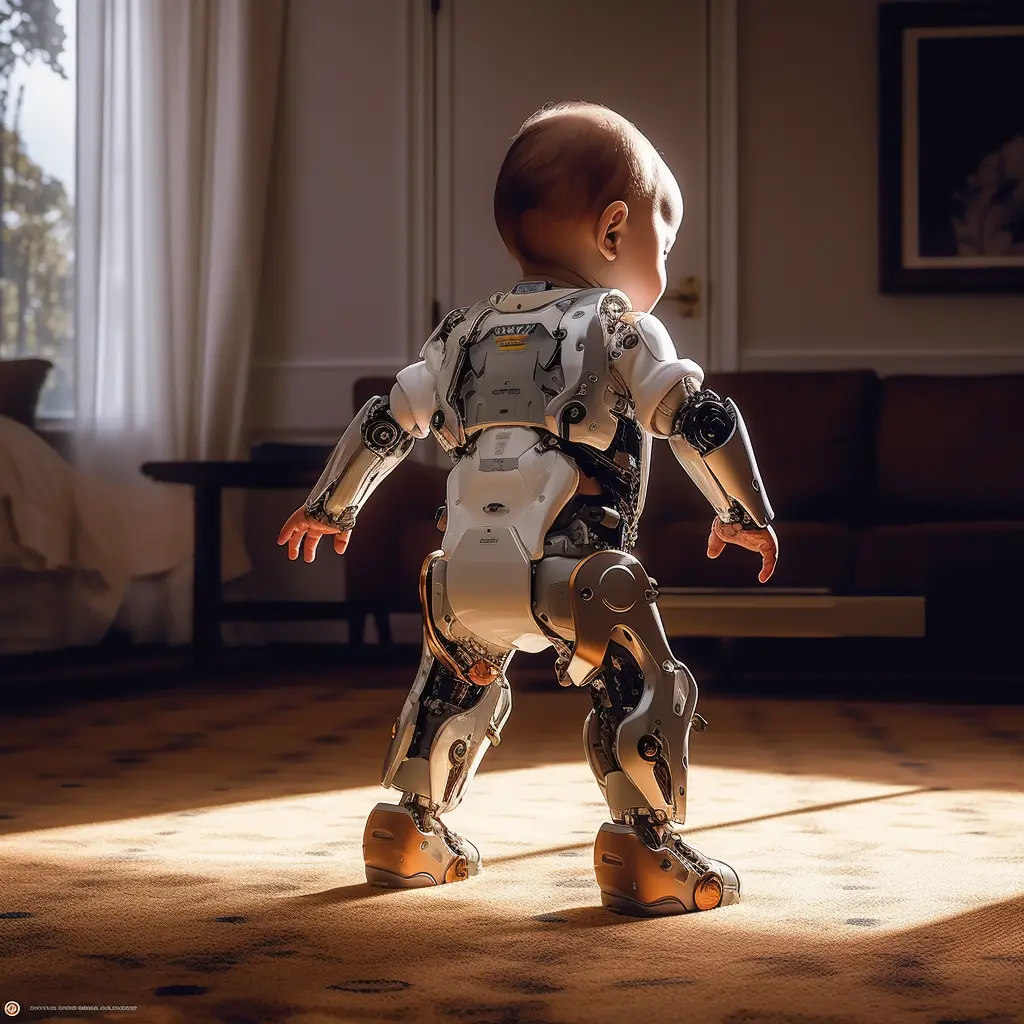
Reinforcement Learning in Action
Reinforcement learning isn’t just a theoretical concept; it’s being used in real-world applications that are transforming industries and shaping our future.
Take autonomous vehicles, for example. These vehicles need to make complex decisions in real-time, such as when to speed up, when to slow down, when to overtake, and when to avoid obstacles. Reinforcement learning algorithms can learn from millions of miles of driving data, continuously improving their decision-making skills to make driving safer and more efficient.
Another example is recommendation systems, like those used by Netflix or Amazon. These systems need to recommend products or movies that users will like, based on their past behaviour. Reinforcement learning algorithms can learn from the feedback of millions of users to make better and more personalized recommendations.
These are just a few examples of how reinforcement learning is being used in the real world. As technology advances and more data becomes available, we can expect to see reinforcement learning applications in even more areas, from healthcare to finance to climate change.
The Future of Reinforcement Learning
As we look towards the future, the potential of reinforcement learning is vast and exciting. As the late Stephen Hawking once said, “Intelligence is the ability to adapt to change.” This quote perfectly encapsulates the essence of reinforcement learning – its ability to adapt and learn from experience.
One area where reinforcement learning could have a significant impact is personalized education. Imagine a learning system that adapts to each student’s learning style and pace, providing personalized exercises and feedback to maximize learning efficiency. Reinforcement learning algorithms could make this a reality, transforming the way we learn and educate.
In healthcare, reinforcement learning could be used to personalize treatment plans for patients with chronic diseases, learning from each patient’s unique characteristics and responses to different treatments to optimize their health outcomes.
In the realm of climate change, reinforcement learning could help optimize energy usage in smart grids, learning from patterns of supply and demand to reduce energy waste and promote the use of renewable resources.
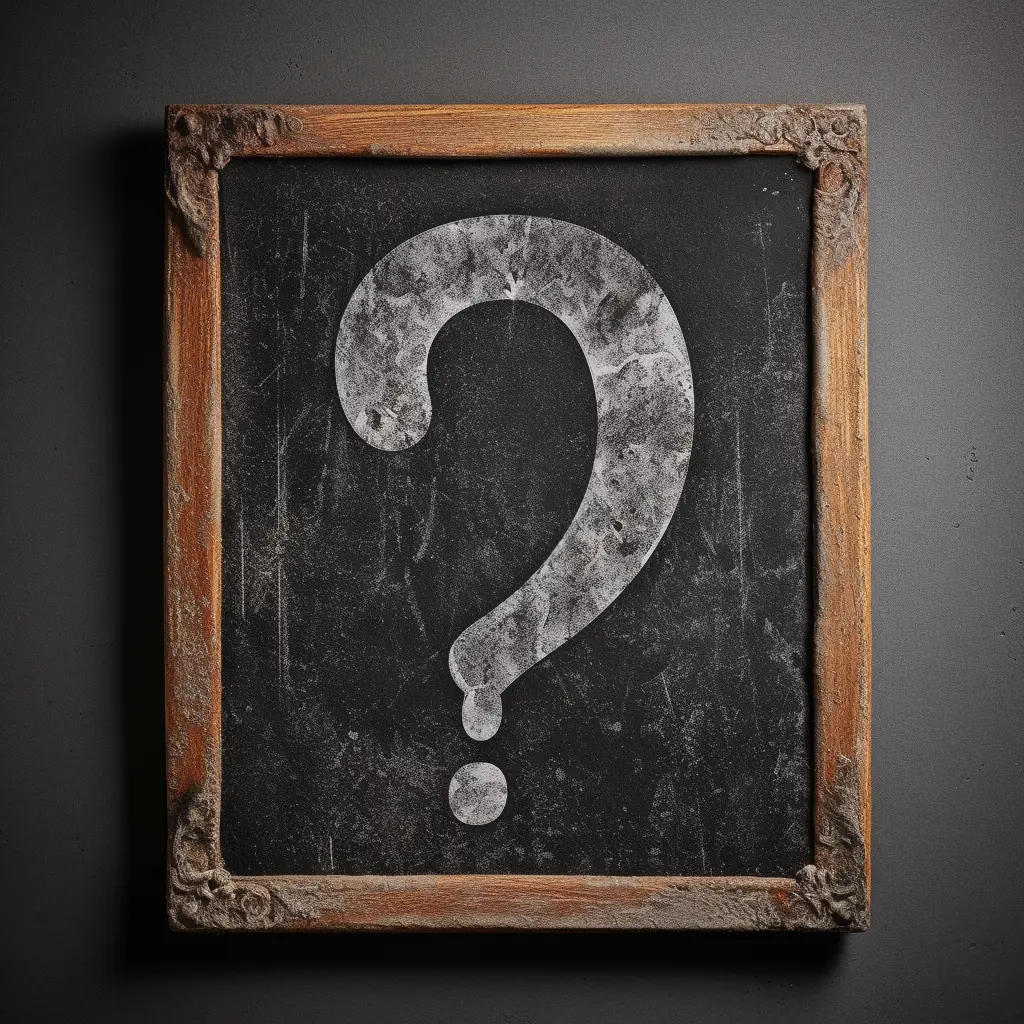
These are just a few of the many ways reinforcement learning could shape our future. But as with any powerful technology, it also raises important questions. How do we ensure that reinforcement learning algorithms make ethical decisions? How do we prevent them from being used maliciously? How do we ensure that the benefits of reinforcement learning are shared equitably across society?
These are the questions we must grapple with as we continue to unravel the future of artificial intelligence.
Conclusion
We’ve embarked on a journey through the world of reinforcement learning, from its basic principles to its controversies, real-world applications, and future potential. We’ve seen how this powerful form of machine learning is already shaping our world and how it could transform our future.
But as we continue to push the boundaries of what machines can learn, we must also grapple with important ethical and societal questions. How will reinforcement learning impact our jobs? How can we ensure it’s used ethically and equitably? What role will we, as humans, play in a world where machines can learn and adapt on their own?
I’ve asked ChatGPT to answer these questions and here’s what it said:
“These are important questions that touch on the ethical and societal implications of reinforcement learning (RL) and AI in general. Here are some thoughts:
- Ensuring Ethical Decisions: The ethical behaviour of RL algorithms largely depends on the reward system and the data they are trained on. To ensure ethical decisions, we need to carefully design the reward system and provide unbiased, representative data for training. It’s also crucial to incorporate ethical guidelines into the design of these algorithms. This might involve interdisciplinary collaboration, bringing together experts in AI, ethics, and related fields. Regular audits and updates based on feedback and societal changes are also necessary.
- Preventing Malicious Use: Preventing the malicious use of RL is a complex issue. It involves not only technical solutions but also legal and regulatory measures. On the technical side, we can implement safeguards and monitoring systems to detect and prevent misuse. On the legal side, we need clear regulations and laws that define acceptable use and provide penalties for misuse. International cooperation might be necessary to effectively regulate the use of RL across borders.
- Equitable Distribution of Benefits: Ensuring that the benefits of RL are shared equitably across society is a significant challenge. It involves addressing issues like the digital divide, data privacy, and algorithmic bias. Education and public awareness about RL and its potential benefits and risks are crucial. Policies should be in place to ensure access to RL technologies and their benefits across different socio-economic groups. Furthermore, efforts should be made to use RL to address societal challenges and improve the quality of life for all, not just a privileged few.
These are complex issues that require ongoing discussion and collaboration among various stakeholders, including AI researchers, ethicists, policymakers, and the public.”
These are open questions and interesting answers that invite further thought and discussion. As we continue to unravel the future of artificial intelligence, one thing is clear: reinforcement learning will play a crucial role in shaping that future.
ChatGPT Notes:
In this engaging collaboration, Manolo and I (ChatGPT) teamed up to craft a comprehensive blog post on the intriguing subject of reinforcement learning in artificial intelligence.
Throughout the process, Manolo’s valuable input and guidance steered our collaboration. This included:
- Initial direction on the blog post’s topic and structure
- Detailed instructions for creating the post, including title suggestions, outline, and introductory text
- Constructive feedback on each section of the draft, leading to several revisions and improvements
- The inclusion of real-world examples, controversial points, and future implications of reinforcement learning
- A request for a thought-provoking conclusion with open-ended questions to stimulate reader engagement
In addition, we decided to use the MidJourney tool to generate captivating, hyper-realistic images to accompany the post, further enhancing its appeal.
This collaborative effort resulted in a blog post that not only educates but also inspires readers about the potential of reinforcement learning in shaping the future of AI.