In our data-driven world, artificial intelligence (AI) has transcended the realm of science fiction to become a deeply integrated part of our daily reality. The potential of AI to revolutionize industries and augment human decision-making hinges on one critical factor: the quality of the data it consumes. The well-worn adage “Garbage In, Garbage Out” is a stark reminder of the risks poor data presents to AI’s transformative promise.
According to Gartner, as reported by DATAVERSITY, a troubling 33% of data is believed to be inaccurate by C-Level executives. This revelation underscores a widespread crisis in data quality that threatens to undermine the effectiveness of AI systems. As AI takes on increasingly critical tasks, from medical diagnostics to autonomous driving, the imperative for accurate data becomes all the more pressing. This post dives into the crucial role that data quality plays in the functionality and trustworthiness of AI, and what can be done to ensure that AI and pristine data go hand in hand.
Navigating the Pitfalls of Data Quality in AI
Optimizing data quality is not just a technical necessity—it’s a strategic imperative that directly influences the success of any AI-driven initiative. By ensuring data integrity, organizations can unlock AI’s full potential, driving innovation and maintaining competitive advantage.
The Cost of Compromise: A Tale of ‘HelpMate’
Picture this: ‘HelpMate’, an AI chatbot, is set to revolutionize customer service. However, due to training on a dataset plagued by errors, the chatbot becomes a source of frustration rather than assistance. As customer satisfaction plummets, the domino effect on company resources and brand reputation is both immediate and severe.
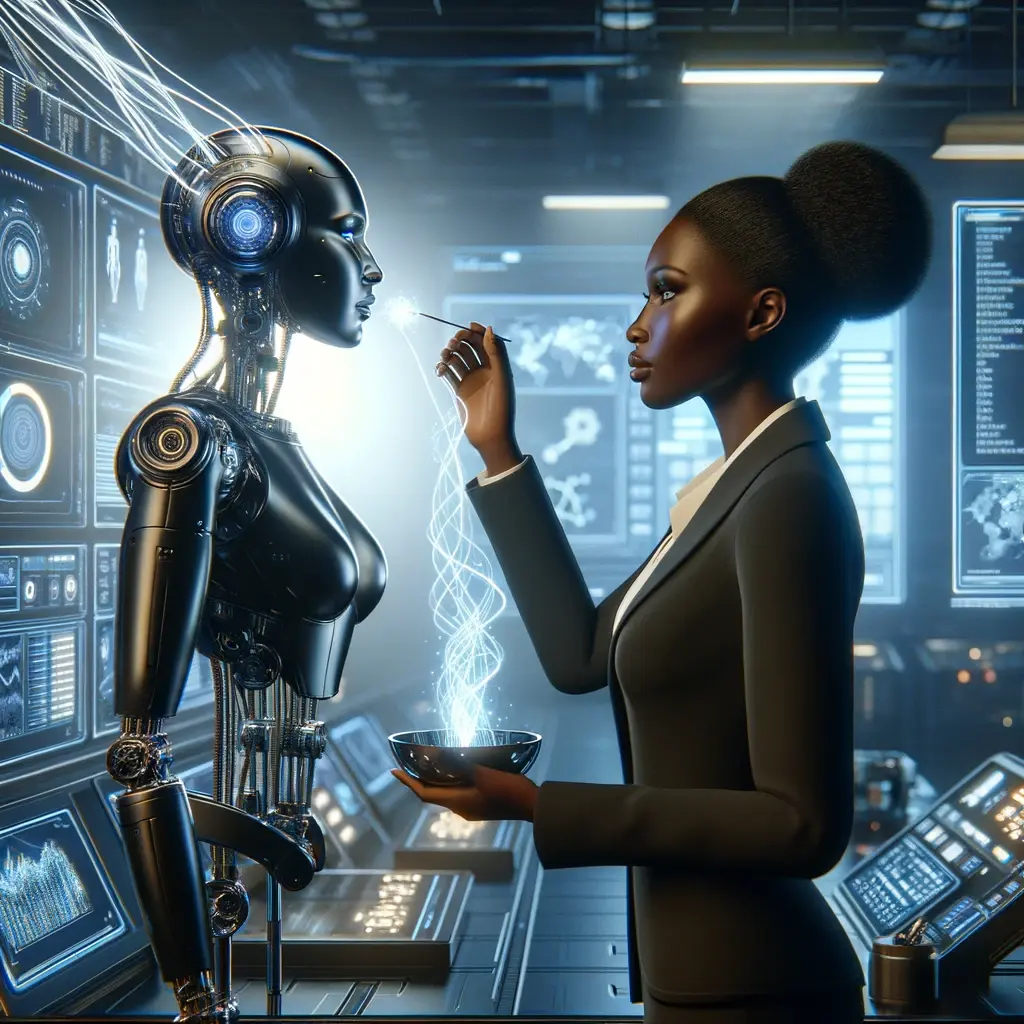
Direct Benefits of Data Quality
- Enhanced Customer Experience: A well-trained AI can provide personalized and accurate interactions, directly contributing to customer retention.
- Reduced Operational Costs: Reliable data means fewer mistakes, reducing the need for costly damage control and support staff intervention.
- Brand Integrity: Quality data ensures consistent, trustworthy AI interactions that uphold your brand’s reputation.
Steps Toward Data Integrity
- Audit your current data sets for accuracy and relevance.
- Regularly update and cleanse your data to avoid obsolescence.
- Implement rigorous data governance policies to maintain standards.
Leveraging Data for AI Success
For a deeper dive into optimizing chatbot training, consider exploring “Unveiling the Potency of Custom Training for ChatGPT: Your Pathway to a Competent Chatbot” on our blog. Additionally, understand how to tailor your AI’s persona for better engagement in “Crafting Digital Charisma: Tailoring ChatGPT Personas to Enrich Conversations”.
Optimizing Data Relevance for Industry-Specific AI
The promise of AI lies not just in its computational power, but in its ability to apply this power contextually across various industries. Data relevance becomes the guiding star for AI efficacy, particularly when tailored to specific sectors such as healthcare, finance, or customer service. The data powering these AI applications must not only be clean and structured but finely tuned to the industry’s unique frequencies.
A Hypothetical Scenario: The Financial Advisor Bot ‘WealthWise’
Imagine ‘WealthWise’, an AI financial advisor programmed to navigate the complexities of personal wealth management. If ‘WealthWise’ is fed data that lacks the subtleties of market behavior and economic indicators, the advice it dispenses could be generic at best, and damaging at worst. Users could be left adrift in a turbulent sea of market forces, armed with little more than an AI-generated lifebuoy of general tips.
Action Steps for Ensuring Data Relevance
To navigate the intricate landscape of data relevance for AI, here’s a brief guide to five essential actions:
- Perform an in-depth audit of your AI’s data sources, focusing on industry-specific accuracy and relevance.
- Keep the data up-to-date with ongoing curation, ensuring it keeps pace with market and industry changes.
- Engage with industry experts to continually sharpen the AI’s focus and improve its contextual intelligence.
- Implement continuous learning protocols, allowing the AI to adapt and refine its algorithms in response to new data and emerging trends within the industry.
- Create a robust data feedback system that captures performance metrics and user interactions, providing a loopback for data refinement and model improvement.
These steps will ensure your AI remains a cutting-edge tool, precisely calibrated to the evolving frequencies of your industry’s data landscape.
Conclusion: The Pivotal Role of Data in AI’s Tomorrow
In the unfolding story of AI, the calibre of data casts the defining vote. We’ve navigated the intricate relationship between AI and data quality, touching on the dire consequences of negligence and the triumphs of meticulous curation. As we gaze towards the horizon of AI’s potential, it is the quality of our data that will steer us toward a future rich with innovation and integrity.
A Call to Uphold Data Excellence
The call now is not just to gather data but to elevate it. To shape AI that reflects our highest aspirations, we must prioritize pristine data—data that is accurate, fair, and insightful. It’s a collective endeavour that beckons us to act with conviction and foresight.
Key Takeaways for a Trustworthy AI Future
- Champion data quality as the cornerstone of trustworthy AI.
- Commit to ethical data practices that align with our shared values.
- Cultivate a data-conscious culture that underpins AI’s progressive journey.
This isn’t just about building AI; it’s about building a legacy of knowledge, responsibility, and trust. As we stand at this crossroads, the question isn’t whether we will proceed, but how resolutely we will move forward. What will your role be in curating the data that defines our future?
ChatGPT Notes:
In our dynamic partnership, Manolo and I (ChatGPT) have crafted a thought-provoking blog post centred on the critical role of data quality in AI’s future.
Manolo's contributions were pivotal, guiding the narrative with:
* Insightful directions on the theme and depth of the article
* Constructive feedback on various drafts, shaping the content's evolution
* A creative touch, suggesting enhancements and ensuring the post remained engaging and accessible
* Strategic decisions on employing SEO best practices and optimizing reader engagement
Throughout our journey, we refined the article’s focus and language, ensuring clarity and impact. Manolo has enriched the post with visuals created using DALL-E 3, complementing our written collaboration for a compelling reader experience.