Artificial intelligence (AI) has become a cornerstone of modern technology, revolutionizing industries and transforming everyday life. From personalized recommendations on streaming platforms to sophisticated healthcare diagnostics, AI’s influence is pervasive and growing. However, with great power comes great responsibility, and the rapid adoption of AI technologies has brought significant privacy risks to the forefront.
Understanding these privacy risks is crucial in our increasingly digital world. As AI systems become more integrated into our daily routines, they collect, analyze, and sometimes share vast amounts of personal data. This data can be sensitive, and its mishandling can lead to severe consequences for individuals and society. In this blog post, we will delve into the top ten AI privacy risks and explore practical strategies to mitigate them, ensuring that we can enjoy the benefits of AI while safeguarding our privacy.
The Paradox of Privacy and New Technology
With each new technological advancement, we seem to edge closer to a world where privacy is a relic of the past. In the quest for convenience and innovation, we often trade away pieces of our personal lives. This paradox is especially evident with AI-driven technologies. We are no longer just being observed; we have become the observers, providing a constant stream of private data to tech giants like Google, Apple, Microsoft and Meta.
These companies have built extensive ecosystems around our data. Every interaction, location, and preference is logged, analyzed, and used to refine algorithms that predict our behavior and needs. While this leads to highly personalized experiences, it also means we are under continuous surveillance, albeit indirectly.
Mesh Networks and Location Tracking
One of the most pervasive methods of data collection is through mesh networks created by our mobile devices. These networks allow devices to communicate with each other without relying solely on central internet connections. While useful for improving connectivity and services, they pose significant privacy risks.
Mobile devices constantly share location data, not just of the user but of anyone who comes into close proximity. This means that our movements can be tracked, and patterns can be inferred, often without our explicit consent. This data can reveal intimate details about our lives, from our daily routines to our personal relationships.
Risks Associated with Mesh Networks
- Unintended Surveillance: By constantly pinging nearby devices, we inadvertently create a map of where we and others go.
- Data Aggregation: Companies can aggregate this data to create detailed profiles, which can be used for targeted advertising or worse, surveillance.
- Security Vulnerabilities: These networks can be exploited by malicious actors to gain access to personal data or track individuals without their knowledge.
Mitigation Strategies
- Limit Location Sharing: Adjust the privacy settings on your mobile devices to limit the sharing of location data.
- Review App Permissions: Regularly review and manage the permissions granted to apps, ensuring they only have access to necessary data.
- Awareness and Education: Stay informed about how your data is being used and take proactive steps to protect your privacy.
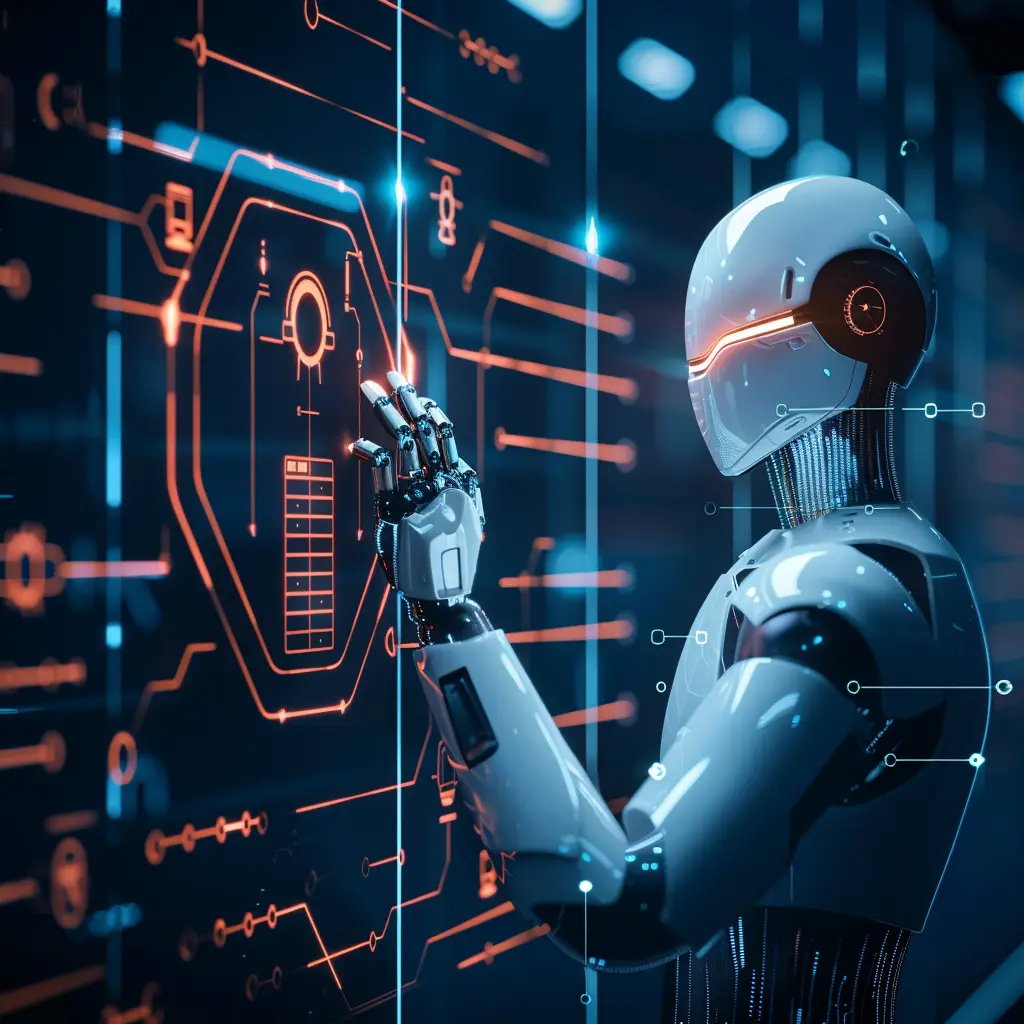
Unauthorized Use of User Data
As AI technologies advance, so does the potential for misuse of user data. One of the most significant privacy risks is the unauthorized use of sensitive information. AI systems can inadvertently expose user data without consent, leading to breaches of privacy and trust.
Example: Apple and ChatGPT
A prominent example of this risk is Apple’s recent decision to restrict employee use of ChatGPT due to concerns about data leakage. However, there are also rumors that Apple is planning to integrate ChatGPT to upgrade Siri, its voice assistant. This paradox highlights a critical issue: while Apple acknowledges the risks associated with data leakage, it appears less concerned when the data potentially exposed belongs to users rather than employees, especially if it can enhance their AI capabilities.
Currently, iPhones are considered among the most secure phones on the market. However, this security does not necessarily equate to superior user privacy. With the integration of more powerful AI like ChatGPT, the tension between security and privacy is likely to increase.
Mitigation Strategies
- Strict Data Access Controls: Implementing robust access controls ensures that only authorized individuals can access sensitive data. This involves using role-based access controls (RBAC) and regularly auditing access logs.
- User Consent Mechanisms: Obtaining explicit user consent before collecting or using their data is crucial. This can be achieved through clear and transparent privacy policies, as well as opt-in mechanisms for data collection.
- Data Anonymization: Transforming personal data into anonymous data helps protect user privacy while still allowing AI models to learn and improve. Techniques like pseudonymization and encryption can be employed to ensure data privacy.
- Regular Audits and Monitoring: Conducting regular audits and monitoring AI systems for unauthorized data use can help detect and prevent potential breaches. This involves continuous monitoring of data flows and usage patterns.
- Privacy-Focused Tools: Using privacy-focused search engines like Startpage and browsers like Tor can help users minimize data collection and enhance their online privacy.
- Encrypted Communication: Use apps like Signal for encrypted peer-to-peer communication, ensuring that your messages are secure and private.
- Open-Source Mobile OS: Consider using open-source mobile operating systems like CalyxOS (calyxos.org) that prioritize privacy and give you greater control over your data.
- Privacy-Focused Apps: Choose apps and services that are transparent about their data practices and committed to protecting user privacy.
- Use of VPNs: Employing virtual private networks (VPNs) can add an extra layer of security, masking your IP address and encrypting your internet traffic to protect your online activities from prying eyes.
- Multiple Browsers for Different Activities: Use separate browsers for different activities, such as one for Google services and social media, and another for general web browsing without logging into any accounts. This helps compartmentalize your online presence and reduces the risk of cross-tracking. I suggest checking brave.com
- Multiple Email Addresses: Utilize multiple email addresses for different purposes (e.g., personal, work, subscriptions) to minimize the amount of data linked to a single account. ProtonMail is one of the best way to get prive email addresses.
- Multiple Mobile Numbers: Maintain multiple mobile numbers to segregate personal and professional communications, reducing the amount of information tied to a single identifier.
- Two Mobile Phones: Consider using two mobile phones, one exclusively for specific services like Google and Facebook, and the other for general use. iPhones, while secure, are difficult to prevent from tracking you entirely, even with opt-out options even if turned off. Therefore, using an iPhone as a secondary device can help manage tracking risks.
By implementing these strategies, organizations and individuals can significantly reduce the risk of unauthorized data use and build trust with their users.
Prompt Injection Attacks
Prompt injection attacks are a sophisticated form of cyberattack that targets AI models by manipulating the input prompts they receive. These attacks can be particularly dangerous because they exploit the model’s inherent design to extract sensitive information or cause unintended behaviors.
How Prompt Injection Attacks Work
Attackers craft malicious prompts designed to deceive AI models into revealing sensitive data or performing actions they are not supposed to. For instance, an attacker might input a seemingly harmless query that, due to the model’s underlying structure, elicits a response containing confidential information.
Example: Exploiting AI Chatbots
An attacker might use a carefully structured question to trick an AI chatbot into disclosing personal information about other users or internal system details. This type of attack highlights the vulnerabilities in AI systems that rely heavily on natural language processing.
Mitigation Strategies
- Robust Input Validation: Implementing stringent input validation processes can help filter out malicious prompts before they reach the AI model. This involves checking the input for known patterns of malicious behavior and sanitizing it accordingly.
- Monitoring and Logging: Continuously monitor and log interactions with AI models to detect and respond to suspicious activities in real-time. Anomaly detection systems can alert administrators to potential prompt injection attacks.
- Limiting Model Outputs: Restrict the types of outputs that AI models can generate, especially when handling sensitive information. Implementing guardrails can prevent models from divulging unauthorized information.
- User Training and Awareness: Educate users and administrators about the risks of prompt injection attacks and best practices for interacting with AI systems. Awareness can help reduce the likelihood of successful attacks.
Insecure Output Handling
Insecure output handling refers to the improper management of data generated by AI models, which can lead to privacy breaches and other security issues. Ensuring that AI outputs are securely managed is crucial for maintaining data integrity and user trust.
Risks of Insecure Output Handling
- Data Breaches: If AI outputs are not properly secured, they can be intercepted or accessed by unauthorized parties, leading to data breaches.
- Sensitive Information Exposure: AI models might inadvertently generate outputs that contain sensitive information, which, if not handled securely, can be exposed to unintended audiences.
Example: Healthcare AI Systems
In healthcare, AI systems often generate outputs that include patient data. If these outputs are not securely managed, they can be exposed, leading to severe privacy violations and potential legal repercussions.
Mitigation Strategies
- Secure Storage: Ensure that AI outputs are stored in secure, encrypted environments. This prevents unauthorized access and ensures that data remains confidential.
- Access Controls: Implement strict access controls to regulate who can view and handle AI-generated outputs. Use role-based access controls (RBAC) to ensure that only authorized personnel have access.
- Output Validation: Validate the data generated by AI models to ensure it does not contain sensitive or confidential information that should not be exposed. This involves implementing checks and filters to identify and manage sensitive data.
- Regular Audits: Conduct regular audits of AI outputs to ensure they are being handled securely and comply with data protection regulations. Audits can help identify potential vulnerabilities and areas for improvement.
Predictive Harms
How Predictive Harms Occur
AI models trained on large datasets can identify patterns and correlations that might not be immediately apparent. For example, an AI could predict a person’s sexual orientation based on their social media activity, or it could infer political views from their browsing history. These predictions can expose sensitive information that individuals might prefer to keep private.
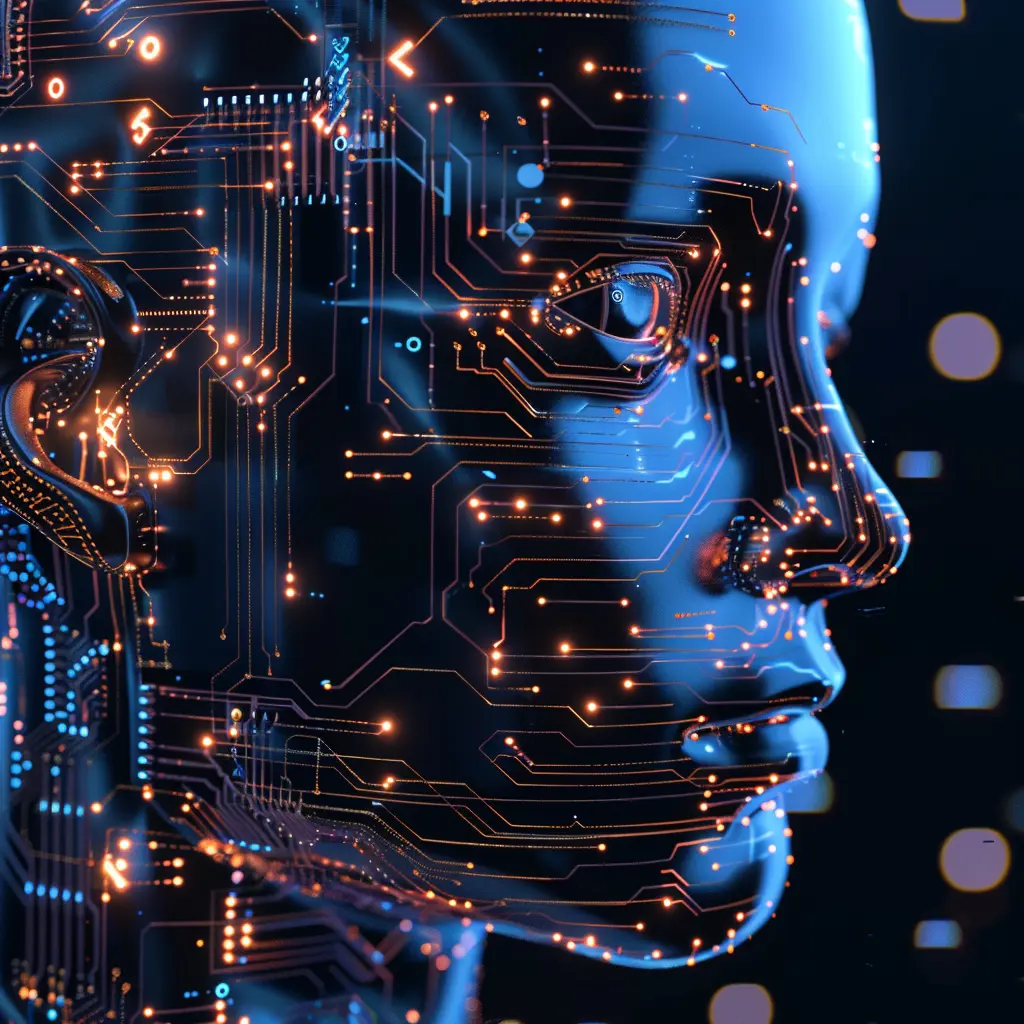
Example: Targeted Advertising
Companies use predictive analytics to deliver highly targeted advertisements. While this can improve user experience, it can also lead to situations where sensitive personal information is inferred and used without explicit consent. For instance, a person might receive ads related to health conditions they have not publicly disclosed, which can be both intrusive and embarrassing.
Mitigation Strategies
- Limiting Scope of Analytics: Restrict the types of predictions AI models are allowed to make, particularly those involving sensitive personal attributes. This can help prevent unintended privacy violations.
- Data Anonymization: Ensure that data used for predictive analytics is anonymized to protect individual identities. Anonymized data reduces the risk of sensitive information being linked back to specific individuals.
- Transparency and Consent: Clearly communicate to users how their data will be used and obtain explicit consent before making predictive inferences. Transparency builds trust and allows users to make informed decisions about their data.
- Ethical AI Practices: Implement ethical guidelines for AI development and use, ensuring that predictive analytics are conducted responsibly and with respect for user privacy.
Group Privacy Violations
AI’s ability to detect patterns in large datasets can lead to group privacy violations, where data about individuals is aggregated and used to make assumptions about entire groups. This can result in stereotyping and discrimination against certain populations.
How Group Privacy Violations Occur
When AI systems analyze data from numerous individuals, they can identify trends and patterns that apply to specific groups. These insights can then be used in ways that negatively impact those groups, such as in hiring practices, loan approvals, or law enforcement activities.
Example: Algorithmic Discrimination
AI algorithms used in hiring processes might inadvertently favor certain demographics over others, leading to discriminatory practices. For instance, if an AI model is trained on data from a predominantly male workforce, it might develop a bias against female candidates, perpetuating gender inequality.
Mitigation Strategies
- Bias Detection and Mitigation: Implement tools and processes to detect and mitigate biases in AI models. Regularly test AI systems to ensure they do not perpetuate stereotypes or discrimination.
- Diverse Training Data: Use diverse and representative datasets to train AI models. This helps ensure that AI systems do not develop biases based on unrepresentative data samples.
- Fairness and Accountability: Establish fairness and accountability standards for AI development and deployment. Ensure that AI systems are audited for compliance with these standards.
- User Feedback and Participation: Encourage feedback from affected groups and involve them in the development and evaluation of AI systems. This helps ensure that AI technologies are fair and inclusive.
Autonomy Harms
AI’s ability to derive information and influence behavior without individuals’ knowledge or consent can lead to autonomy harms. These occur when AI systems manipulate individuals’ decisions and actions, undermining their autonomy.
How Autonomy Harms Occur
AI systems can be designed to subtly influence user behavior, such as through personalized recommendations or targeted advertising. While often intended to enhance user experience, these manipulations can infringe on personal autonomy, making individuals act in ways they might not have chosen independently.
Example: Behavioral Targeting
Social media platforms use AI to personalize content and advertisements, encouraging users to spend more time on their sites. This can lead to addictive behaviors and influence users’ choices, such as purchasing decisions or political views, without their conscious awareness.
Mitigation Strategies
- Transparency in AI Operations: Clearly explain how AI systems influence user behavior and provide options for users to opt out of such influence. Transparency helps users understand and control their interactions with AI.
- User Control and Consent: Ensure that users have control over the extent to which AI systems can influence their decisions. Obtain explicit consent before using AI to manipulate user behavior.
- Ethical AI Design: Design AI systems with ethical considerations in mind, prioritizing user autonomy and informed decision-making. Avoid creating AI technologies that manipulate users for profit or other interests.
- Regular Audits and Reviews: Conduct regular audits of AI systems to ensure they adhere to ethical standards and do not infringe on user autonomy. Involve independent reviewers to assess compliance with ethical guidelines.
Excessive Agency
Excessive agency in AI systems refers to scenarios where AI operates with too much autonomy, making decisions and taking actions without adequate human oversight. This can lead to misuse, errors, and unintended consequences that undermine user trust and safety.
How Excessive Agency Occurs
AI systems with excessive agency are designed to perform tasks independently, sometimes even self-policing or modifying their own parameters. Without human intervention, these systems can make flawed decisions or behave unpredictably, especially in complex or dynamic environments.
Example: Autonomous Weapons Systems
A prominent example of the risks associated with excessive agency is autonomous weapons systems. These systems, designed to make lethal decisions without human intervention, pose significant ethical and safety concerns. The potential for errors or misuse in such high-stakes scenarios underscores the dangers of granting AI excessive autonomy.
Mitigation Strategies
- Human-in-the-Loop Systems: Ensure that AI systems have human oversight and intervention capabilities. Critical decisions, especially those involving safety or ethical considerations, should always involve human judgment.
- Clear Accountability Frameworks: Establish clear accountability frameworks that define who is responsible for the actions and decisions made by AI systems. This helps ensure that AI developers and operators remain answerable for the behavior of their systems.
- Robust Testing and Validation: Conduct thorough testing and validation of AI systems to ensure they operate reliably and safely within their intended parameters. This includes stress-testing systems in various scenarios to identify potential failures.
- Ethical Guidelines and Policies: Develop and enforce ethical guidelines and policies that limit the autonomy of AI systems. These guidelines should emphasize the importance of human oversight and the prevention of harm.
Conclusion
The integration of AI into our daily lives offers tremendous benefits, but it also brings significant privacy risks. Understanding these risks and implementing effective mitigation strategies is crucial to safeguard our personal information and maintain trust in AI technologies.
From unauthorized use of user data to the dangers of excessive agency, each privacy risk requires a tailored approach to mitigation. By adopting robust security measures, ethical AI practices, and privacy-focused tools, we can navigate the complex landscape of AI privacy.
In our quest for convenience, we have often traded away our privacy. As technology becomes more ingrained in our lives, reclaiming that privacy has become increasingly difficult and expensive. We are on a trend that is challenging to reverse. However, there is hope. The development of open-source AI that prioritizes user privacy and defends our interests could be a game-changer. Such technologies can help ensure that AI serves to enhance our lives without compromising our privacy.
Starting today, we must take steps to protect our privacy. We should support open-source software, privacy-focused tools, and open-source AI solutions. These tools are essential in defending our identity and privacy.
“Technology should improve your life, not become your life.” – Billy Cox
Open Questions
- How can we balance AI innovation with privacy protection?
- What role should governments play in regulating AI privacy?
- How can individuals take control of their data in the AI era?
By exploring these questions, we can continue the conversation on AI privacy and work towards solutions that benefit everyone.
ChatGPT Notes
In this interactive collaboration, Manolo and I (ChatGPT) created an in-depth blog post on understanding and mitigating AI privacy risks.
- Manolo provided initial guidance on the blog topic and audience.
- We crafted a detailed outline and refined it based on his feedback.
- I incorporated Manolo’s suggestions, including real-life examples and additional mitigation strategies.
- We added insights on open-source tools and expanded the conclusion.
- Images accompanying the post were generated using MidJourney.
This collaboration ensured a comprehensive and educational blog post.